GPU Aggregators play a crucial role in the market by consolidating GPU supply, simplifying networking orchestration, and enhancing the overall experience with ML-specific software. These platforms essentially act as intermediaries, similar to Web2 VARs, providing a range of value-added services to users. By offering comprehensive GPU solutions that support various tasks such as model training, fine-tuning, and inference, GPU aggregators streamline the process for users in need of computational resources.
One pioneering protocol in this space is Io.net, which has paved the way for efficient GPU distribution and management. However, as the demand for consolidated GPU resources continues to grow, it is anticipated that more competitors will enter the market to meet the evolving needs of users. This competition is likely to drive innovation, leading to the development of new and improved GPU aggregation protocols that offer enhanced features and capabilities.
In today’s dynamic digital landscape, where machine learning and AI applications are becoming increasingly prevalent, the role of GPU aggregators is becoming more significant. These platforms not only simplify access to GPU resources but also contribute to the optimization of ML workflows by providing tailored solutions that cater to specific requirements. As the market for GPU aggregation continues to expand, users can expect a wider range of options and enhanced functionalities that will further streamline their machine learning processes.
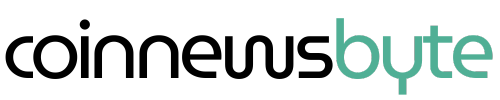